- publication
- IEEE International Conference of Computer Vision Workshops 2015
- authors
- Kaan Yücer, Oliver Wang, Alexander Sorkine-Hornung, Olga Sorkine-Hornung
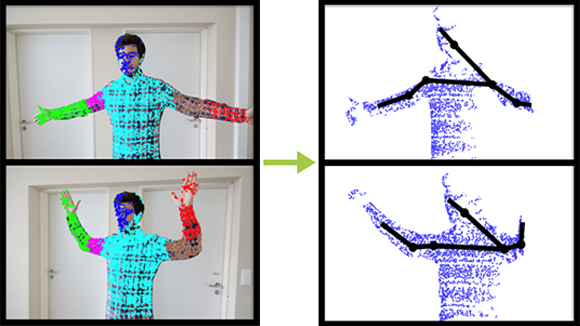
Our technique automatically reconstructs articulated objects from a single video taken by a moving camera. On the left, we show automatically computed segmentations of the articulated object into its piecewise-rigid components. These segmentations are then used to compute the 3D shape and motion of the articulated object at each frame (right), making use of kinematic constraints applied on the skeletal joints of the object.
abstract
Many scenes that we would like to reconstruct contain articulated objects, and are often captured by only a single, non-fixed camera. Existing techniques for reconstructing articulated objects either require templates, which can be challenging to acquire, or have difficulties with perspective effects and missing data. In this paper, we present a novel reconstruction pipeline that first treats each feature point tracked on the object independently and incrementally imposes constraints. We make use of the idea that the unknown 3D trajectory of a point tracked in 2D should lie on a manifold that is described by the camera rays going through the tracked 2D positions. We compute an initial reconstruction by solving for latent 3D trajectories that maximize temporal smoothness on these manifolds. We then leverage these 3D estimates to automatically segment an object into piecewise rigid parts, and compute a refined shape and motion using sparse bundle adjustment. Finally, we apply kinematic constraints on automatically computed joint positions to enforce connectivity between different rigid parts, which further reduces ambiguous motion and increases reconstruction accuracy. Each step of our pipeline enforces temporal smoothness, and together results in a high quality articulated object reconstruction. We show the usefulness of our approach in both synthetic and real datasets and compare against other non-rigid reconstruction techniques.
downloads
- Paper (ICCVW 2015, official version available at www.cv-foundation.org)
- Supplemental
- Video
- BibTex entry
accompanying video
acknowledgments
We are grateful to Joel Bohnes for helping with the implementation and Maurizio Nitti for generating the Robot data set. We thank Ijaz Akhter, Lourdes Agapito, Joao Fayad, Yuchao Dai and Jingyu Yan for sharing their code and data with us. Lastly, we thank the authors of [4,16,23,24,26] for making their code and data sets available online.