- publication
- NeurIPS 2021
- authors
- Amir Hertz, Or Perel, Raja Giryes, Olga Sorkine-Hornung, Daniel Cohen-Or
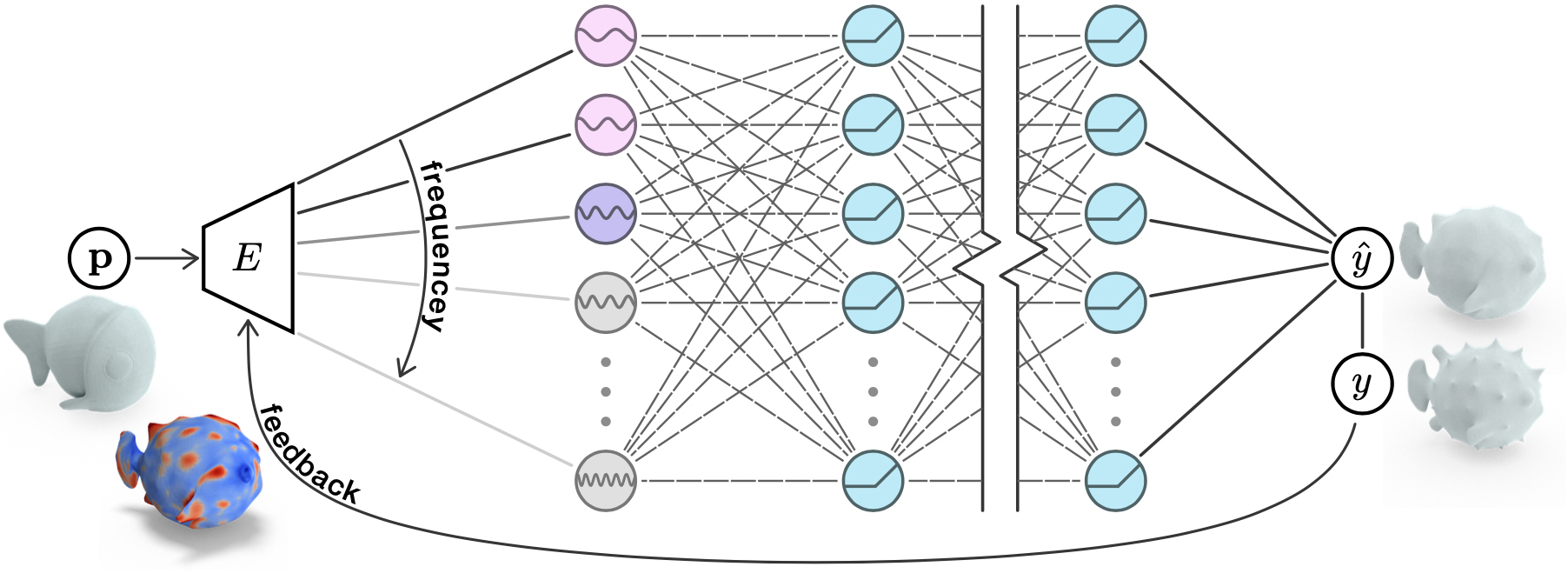
abstract
Multilayer-perceptrons (MLP) are known to struggle with learning functions of high-frequencies, and in particular cases with wide frequency bands. We present a spatially adaptive progressive encoding (SAPE) scheme for input signals of MLP networks, which enables them to better fit a wide range of frequencies without sacrificing training stability or requiring any domain specific preprocessing. SAPE gradually unmasks signal components with increasing frequencies as a function of time and space. The progressive exposure of frequencies is monitored by a feedback loop throughout the neural optimization process, allowing changes to propagate at different rates among local spatial portions of the signal space. We demonstrate the advantage of SAPE on a variety of domains and applications, including regression of low dimensional signals and images, representation learning of occupancy networks, and a geometric task of mesh transfer between 3D shapes.
downloads
acknowledgments
We would like to thank Hao (Richard) Zhang, Hadar Averbuch-Elor and Rinon Gal for their insightful comments, and the anonymous reviewers for their helpful remarks. This work was supported in part by the Israel Science Foundation grant no. 2492/20 and by the ERC-StG grant no. 757497 (SPADE).