- publication
- SIGGRAPH 2024 (conference paper)
- authors
- Peizhuo Li, Sebastian Starke, Yuting Ye, Olga Sorkine-Hornung
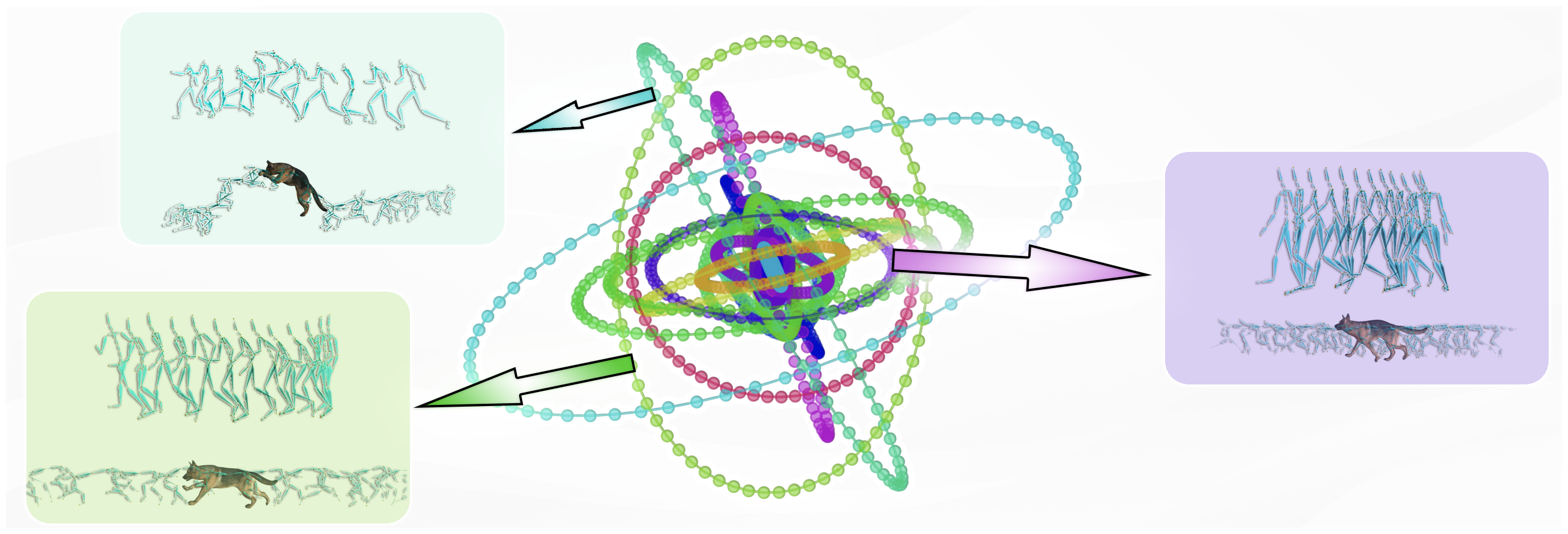
abstract
We present a new approach for understanding the periodicity structure and semantics of motion datasets, independently of the morphology and skeletal structure of characters. Unlike existing methods using an overly sparse high-dimensional latent, we propose a phase manifold consisting of multiple closed curves, each corresponding to a latent amplitude. With our proposed vector quantized periodic autoencoder, we learn a shared phase manifold for multiple characters, such as a human and a dog, without any supervision. This is achieved by exploiting the discrete structure and a shallow network as bottlenecks, such that semantically similar motions are clustered into the same curve of the manifold, and the motions within the same component are aligned temporally by the phase variable. In combination with an improved motion matching framework, we demonstrate the manifold’s capability of timing and semantics alignment in several applications, including motion retrieval, transfer and stylization.
downloads
video
acknowledgments
We thank the anonymous reviewers for their valuable feedback. We also thank Heyuan Yao and Alexander Winkler for the insightful discussions. This work was supported in part by the ERC Consolidator Grant No. 101003104 (MYCLOTH).