- publication
- CVPR 2020 (oral)
- authors
- Wang Yifan, Noam Aigerman, Vladimir G. Kim, Siddhartha Chaudhuri, Olga Sorkine-Hornung
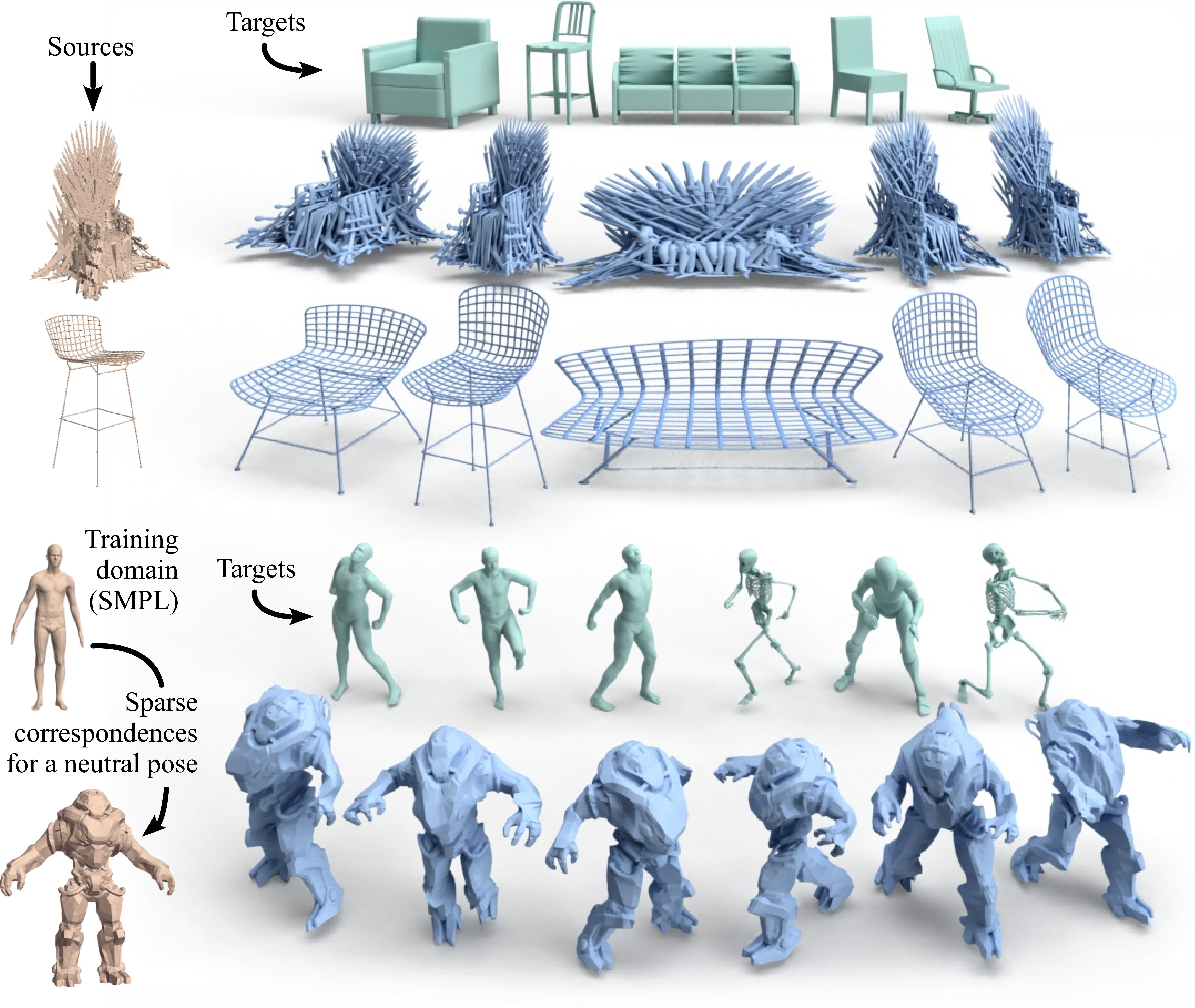
Applications of our neural cage-based deformation method. Top: Complex source chairs (brown) deformed (blue) to match target chairs (green), while accurately preserving detail and style with non-homogeneous changes that adapt different regions differently. No correspondences are used at any stage. Bottom: A cage-based deformation network trained on many posed humans (SMPL) can transfer various poses of novel targets (SCAPE, skeleton, X-Bot, in green) to a very dissimilar robot of which only a single neutral pose is available. A few matching landmarks between the robot and a neutral SMPL human are required. Dense correspondences between SMPL humans are used only during training.
abstract
We propose a novel learnable representation for detail-preserving shape deformation. The goal of our method is to warp a source shape to match the general structure of a target shape, while preserving the surface details of the source. Our method extends a traditional cage-based deformation technique, where the source shape is enclosed by a coarse control mesh termed \emph{cage}, and translations prescribed on the cage vertices are interpolated to any point on the source mesh via special weight functions. The use of this sparse cage scaffolding enables preserving surface details regardless of the shape's intricacy and topology. Our key contribution is a novel neural network architecture for predicting deformations by controlling the cage. We incorporate a differentiable cage-based deformation module in our architecture, and train our network end-to-end. Our method can be trained with common collections of 3D models in an unsupervised fashion, without any cage-specific annotations. We demonstrate the utility of our method for synthesizing shape variations and deformation transfer.
talk
downloads
acknowledgments
We thank Rana Hanocka and Dominic Jack for their extensive help. The robot model is from SketchFab, licensed under CC-BY. This work was supported in part by gifts from Adobe, Facebook and Snap, Inc.