- publication
- ACM SIGGRAPH Asia 2023 (conference paper)
- authors
- Floor Verhoeven, Tanguy Magne, Olga Sorkine-Hornung
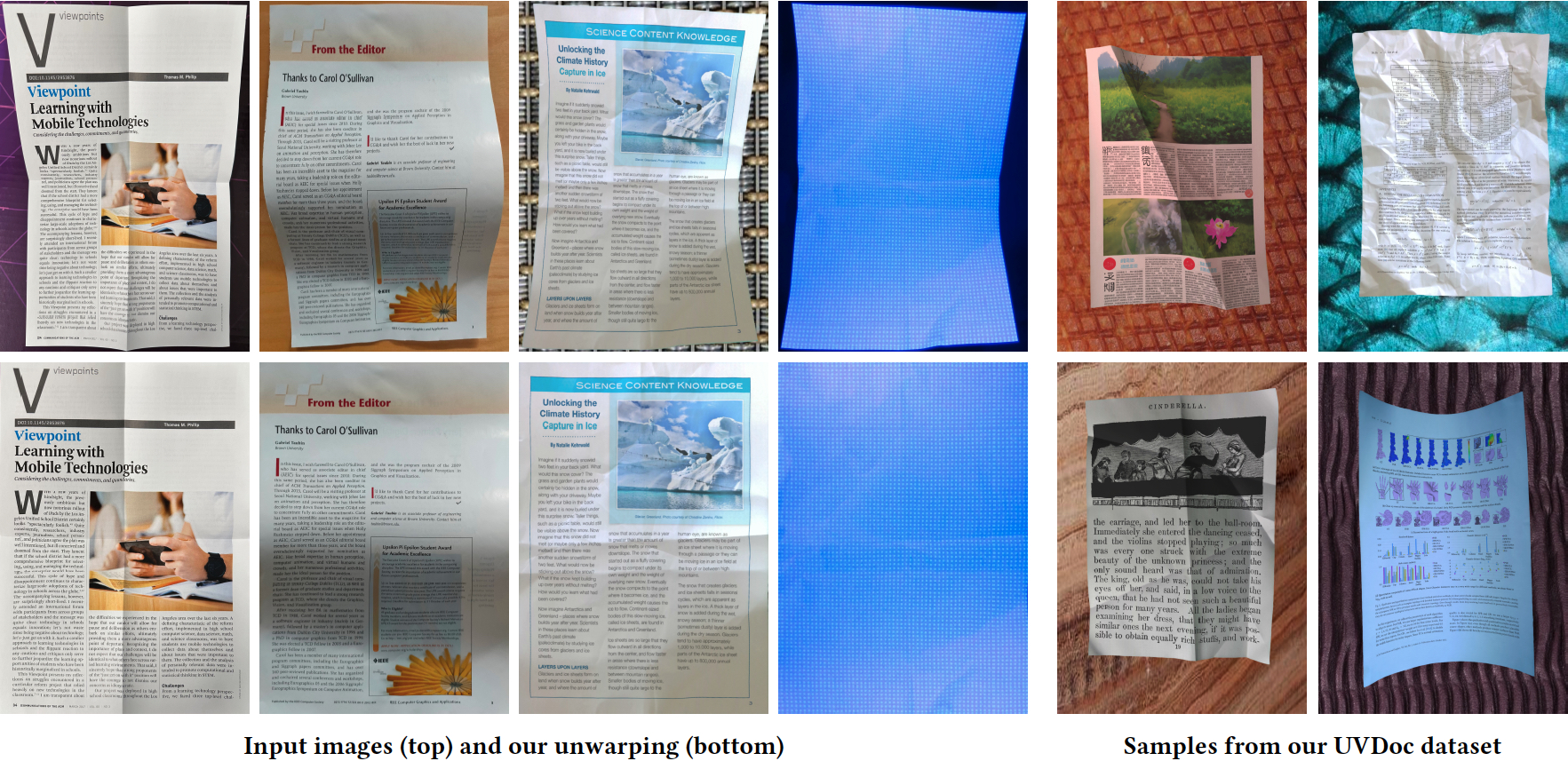
abstract
Restoring the original, flat appearance of a printed document from casual photographs of bent and wrinkled pages is a common everyday problem. In this paper we propose a novel method for grid-based single-image document unwarping. Our method performs geometric distortion correction via a fully convolutional deep neural network that learns to predict the 3D grid mesh of the document and the corresponding 2D unwarping grid in a dual-task fashion, implicitly encoding the coupling between the shape of a 3D piece of paper and its 2D image. In order to allow unwarping models to train on data that is more realistic in appearance than the commonly used synthetic Doc3D dataset, we create and publish our own dataset, called UVDoc, which combines pseudo-photorealistic document images with physically accurate 3D shape and unwarping function annotations. Our dataset is labeled with all the information necessary to train our unwarping network, without having to engineer separate loss functions that can deal with the lack of ground-truth typically found in document in the wild datasets. We perform an in-depth evaluation that demonstrates that with the inclusion of our novel pseudo-photorealistic dataset, our relatively small network architecture achieves state-of-the-art results on the DocUNet benchmark. We show that the pseudo-photorealistic nature of our UVDoc dataset allows for new and better evaluation methods, such as lighting-corrected MS-SSIM. We provide a novel benchmark dataset that facilitates such evaluations, and propose a metric that quantifies line straightness after unwarping.
downloads
acknowledgments
We thank Ruben Schenk for his help with data capture. This work was supported in part by the European Research Council (ERC) under the European Union's Horizon 2020 Research and Innovation Programme (ERC Consolidator Grant, agreement No. 101003104, MYCLOTH).